Today’s agricultural automation lies at the end of a long evolution of mechanization throughout the history of agriculture. The Food and Agriculture Organization of the United Nations (FAO) defines mechanization as the use of all means of machinery and equipment, from simple and basic hand tools to more sophisticated and motorized machinery, in agricultural operations.14 With mechanization, therefore, only the performing part of agricultural work is automated, and the degree of automation increases as we move from basic hand tools towards motorized machinery.
Two phases always precede the performing of any agricultural operation: diagnosis and decision-making. Figure 1 represents the three phases as a cyclical process with continuous feedback between them. The implementation of any agricultural operation – from harvesting, to disease control, to irrigation – generally starts by diagnosing the issue at hand to determine what, if any, action is needed. By way of illustration, before irrigating, producers need to know whether plants require water. Similarly, livestock producers need to know the health status of animals before prescribing antibiotics. A diagnosis can be made using producers’ experience, but it can also be automated through sensors monitored by the producers. Once a diagnosis is made, producers decide what needs to be done (e.g. the amount of irrigation or antibiotics needed) and when. Decisions can then be made by agricultural producers based on their experience and knowledge, or they can be automated by controllers sending signals based on information received from sensors in the diagnosis phase. In the third and final phase (performing), farmers can either conduct agricultural operations directly, using hand tools or animals, or operate various machines. The most advanced automation technologies allow the three phases to be entirely automated. Fruit-harvesting robots are a case in point. These robots carry out all three phases sequentially and automatically, while agricultural producers simply monitor the sensors and maintain the equipment.
FIGURE 1 Three-phase cycle of an automation system
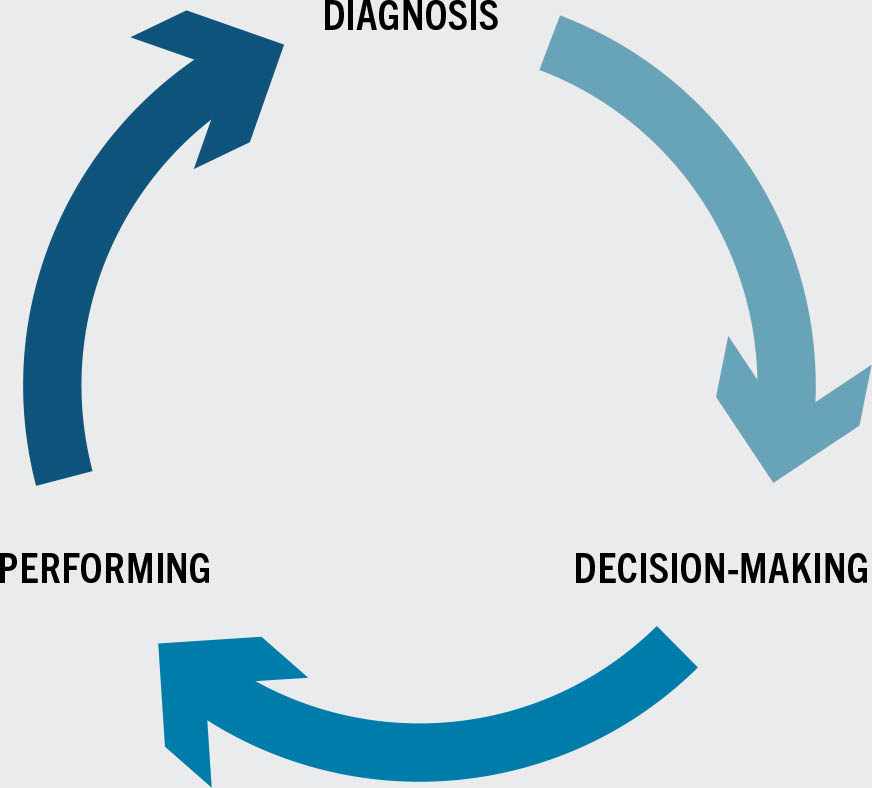
Any technology that automates at least one of the three phases may be classified as an automation technology. Motorized mechanization using engine power15 focuses essentially on the last of the three phases: performing. It automates agricultural operations such as ploughing, seeding, fertilizing, milking, feeding, harvesting and irrigating, among many others. For the purpose of this report, any technology that assists agricultural producers in one or more of the three phases in Figure 1 is considered an automation technology. This includes situations where, for example, agricultural producers use sensors to monitor plants and animals, thus automating the diagnosis phase, but make decisions based on their own experience without the assistance of automated equipment. In some cases, the performing phase can also involve sensing (e.g. the creation of yield maps during harvesting), which then feeds into the diagnosis phase, hence the cyclical representation of Figure 1.
With the rise of digital technologies and automated equipment such as sensors and robots that rely on machine learning and AI, the automation of diagnosis and decision-making becomes possible. Motorized machines are increasingly complemented, or even superseded, by new digital equipment that automates diagnosis and decision-making. For example, a conventional tractor can be converted into an automated vehicle capable of sowing a field autonomously.15 Therefore, while mechanization eases and reduces hard and repetitive work and relieves labour shortages, digital automation technologies further improve productivity by allowing more precise implementation of agricultural operations and more efficient use of resources and inputs. As a consequence, digital automation can lead to gains in environmental sustainability and greater resilience to climate shocks and stresses. However, the possible effects on labour require careful consideration, as explained later in the report.
Figure 2 represents this technological evolution, illustrating the progression of agricultural technologies – with examples of each – from those that assist solely the physical performing of operations to those that assist diagnosis and decision-making. The technological evolution may be summarized through the following technology categories:
- Manual tools, where humans make the diagnosis and the decisions, while the performing is assisted by simple tools, such as axes and hoes.
- Animal traction, where humans still make the diagnosis and the decisions, but physical agricultural operations are performed, or eased, by animals operating agricultural machinery such as ploughs.
- Motorized mechanization, where humans continue to make the diagnosis and the decisions, but motorized machinery and equipment perform the operations. This category marks a shift in the source of energy used on the farm from internal (e.g. human muscle and animals) to external (e.g. fossil fuels and electricity). This shift, however, calls for specific infrastructures to ensure the continuous availability of these energy sources.
- Digital equipment, where a wide range of digital tools assist humans to improve the diagnosis and/or decision-making by automating mental work or by increasing the precision of motorized machinery.
- Robotics with AI, where humans rely on agricultural robots that use AI for all functions of diagnosis, decision-making and performing. These can be static (e.g. milking robots) or mobile (e.g. fruit-harvesting robots). Humans monitor the sensors and maintain the robots. This category includes the most advanced automation technologies, some of which have not yet been scaled up or are still under development.
FIGURE 2 Evolution of agricultural automation
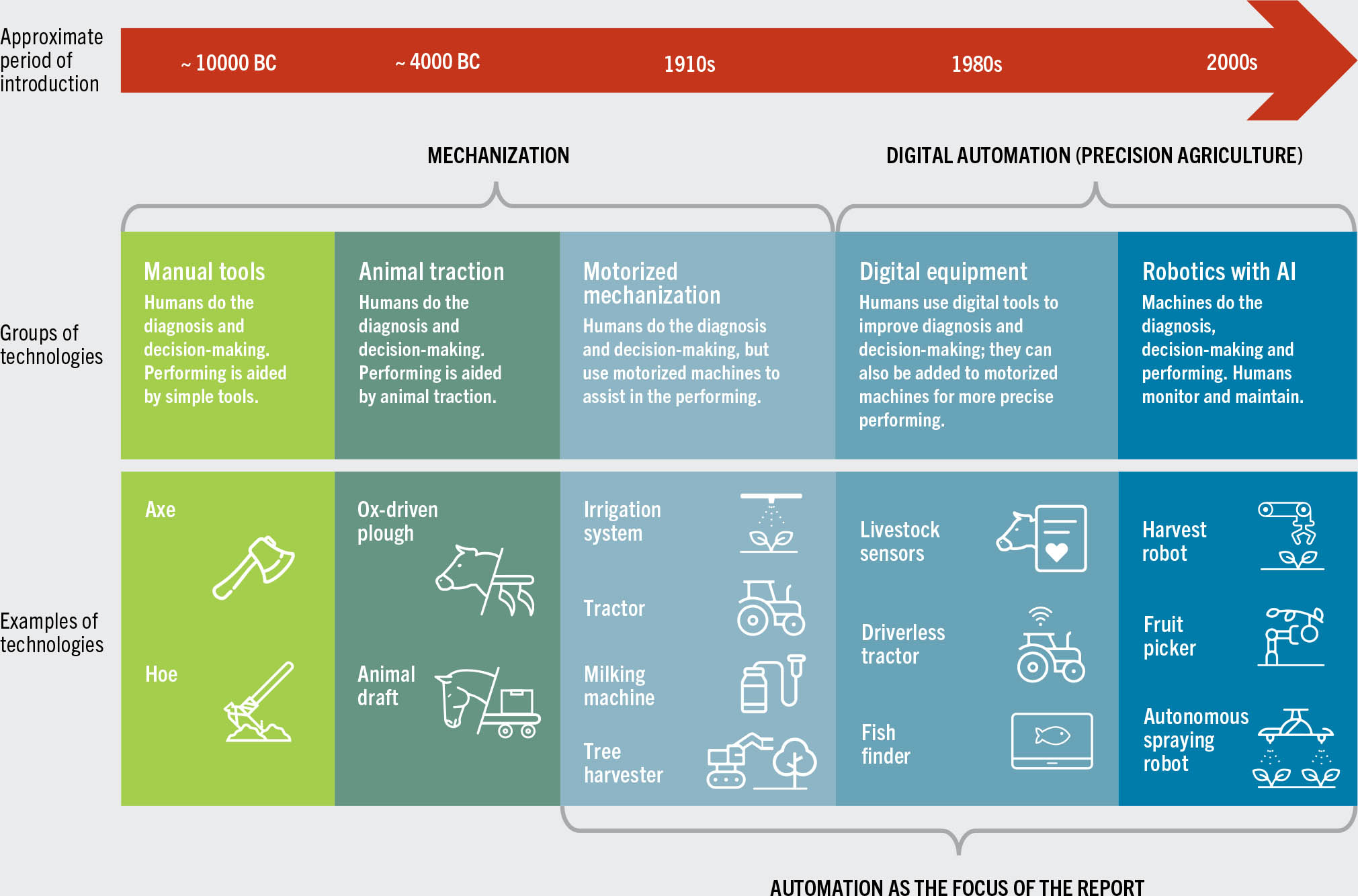
Unfortunately, this variety of tools and technologies has contributed to inconsistent definitions of agricultural automation in the literature, hampering efforts to collect automation data.11 For example, some define agricultural automation as autonomous navigation by robots without human intervention, providing precise information to help develop agricultural operations.16 Others define it as accomplishment of production tasks through mobile, autonomous, decision-making, mechatronic devices.17 However, these definitions are very restrictive and do not capture all the aspects and forms of automation – static equipment, such as robotic milking machines, is a case in point. Moreover, the definitions exclude not only most motorized machinery that automates the performing of agricultural operations, but also digital tools (e.g. sensors) that automate only diagnosis.
Figure 2 is a simplification of the historical reality of the evolution of automation technologies; there can be overlaps and grey areas between the categories. Nevertheless, it helps to outline the focus of this report and define agricultural automation. The concept of agricultural automation is applied to the three blue-shaded boxes, which constitute the focus of the report. On this basis, the report proposes a definition of agricultural automation as:
the use of machinery and equipment in agricultural operations to improve their diagnosis, decision-making or performing, reducing the drudgery of agricultural work and/or improving the timeliness, and potentially the precision, of agricultural operations.
By this definition, agricultural automation includes precision agriculture, which is a management strategy that gathers, processes and analyses data to improve management decisions (see Glossary).
Starting from the first blue-shaded box in Figure 2, motorized mechanization includes machines operated by humans to perform tasks such as ploughing, irrigating and milking. However, humans make the diagnosis based on their own observation or by measuring simple parameters; they then make decisions based on (internal or external) experience, knowledge and available resources. The last two categories of Figure 2 cover digital automation. They include a wide range of tools, equipment and software that are, or can be, multifunctional and interdisciplinary, allowing the management of resources throughout the system to be highly optimized, individualized, intelligent and anticipatory.18 As digital automation technologies (robotics with AI) develop, all three phases – diagnosis, decision-making and performing – can be automated, with the human role largely confined to monitoring and maintenance of automation equipment. This is the case with the fruit picker, for example: when the picking arm receives a message from the controller, based on information from the sensors, it proceeds to pick the fruit.
Automation can involve any one or a combination of the three connected phases. For example, diagnosis may be carried out by sensors while decision-making and performing depend totally on humans. Alternatively, both diagnosis and decision-making may be executed by digital technologies while performing is done by humans. An example of a fully automated system where all three phases are automated is the autonomous spraying robot: the system first obtains data on soil fertility, then decides on the operation area and application rate, and finally applies fertilizer based on that variable rate.