Annex 1. Classification, data sources and regional estimates of agrifood-system employment
Annex Table 1.1 Agrifood-system activities based on the ISIC codes
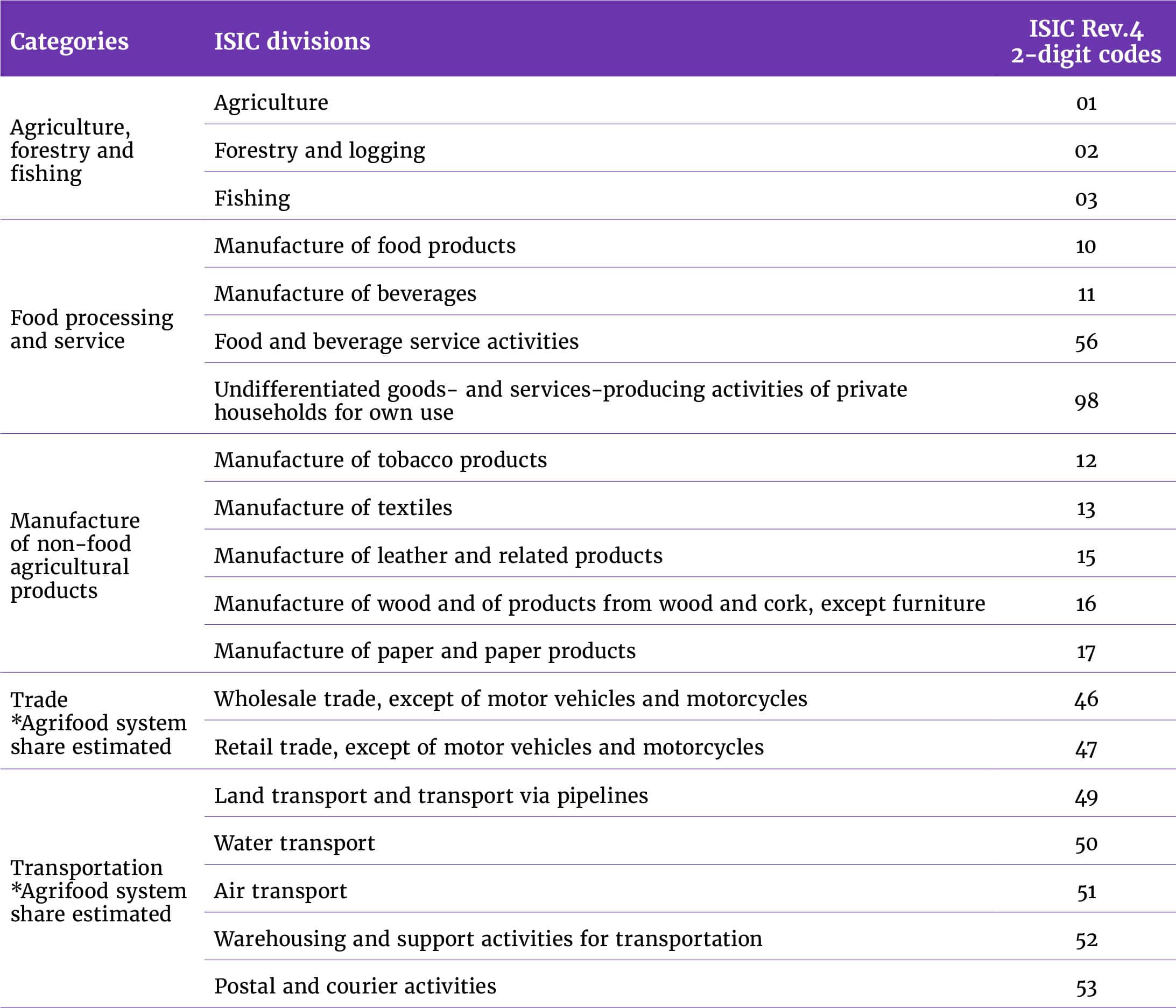
NOTE: *The agrifood systems shares in total trade and transport are estimated using a methodology described in
Davis, B., Mane, E., Gurbuzer, L.Y., Caivano, G., Piedrahita, N., Schneider, K., Azhar, N. et al. 2023.
Estimating global and country-level employment in agrifood systems. FAO Statistics Working Paper Series, No. 23–34. Rome, FAO.
https://doi.org/10.4060/cc4337en Annex Figure 1.1 shows the share of agrifood-system employment in total employment and compares the estimates from the RuLIS1 pool of household surveys with the estimates from the ILOSTAT2 database. The agrifood-system employment estimates may differ between the two sources for three main reasons.2 First, though both databases use household surveys, the survey used for specific countries to compute the agrifood-system employment may differ (for example, living standards measurement surveys versus labour force surveys) in terms of question formulation and sampling etc. Second, the employment figures of some countries included in the ILOSTAT database are computed using the new measure of employment based on the nineteenth International Conference of Labour Statisticians resolution, excluding people whose work was performed for own-use production (for ex. Sierra Leone). Finally, while RuLIS surveys computed employment in AFS using 3–4-digit ISIC codes available in the microdata sets to increase the precision, ILOSTAT disseminated the same data using two-digit ISIC codes. The RuLIS estimates tend to be higher than those from ILOSTAT, but the difference is not great across most countries for which we have data (Annex Figure 1.1). It is, however, more pronounced with respect to measures of women’s employment than with men’s employment.
Annex Figure 1.1 Share of employment in agrifood systems, RuLIS versus ILOSTAT data
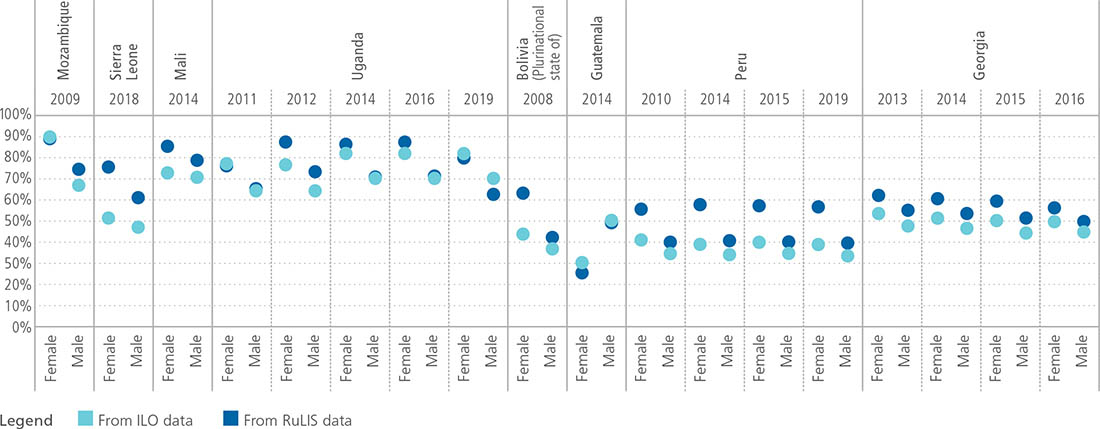
SOURCE: Costa, V., Piedrahita, N., Mane, E., Davis, B., Slavchevska, V. & Gurbuzer, Y. L. (forthcoming). Women’s employment in agrifood systems. Background paper for The status of women in agrifood systems, 2023. Rome, FAO.
Annex Figure 1.2 The share of agrifood-system employment in total employment in 2005 and 2019, by sex
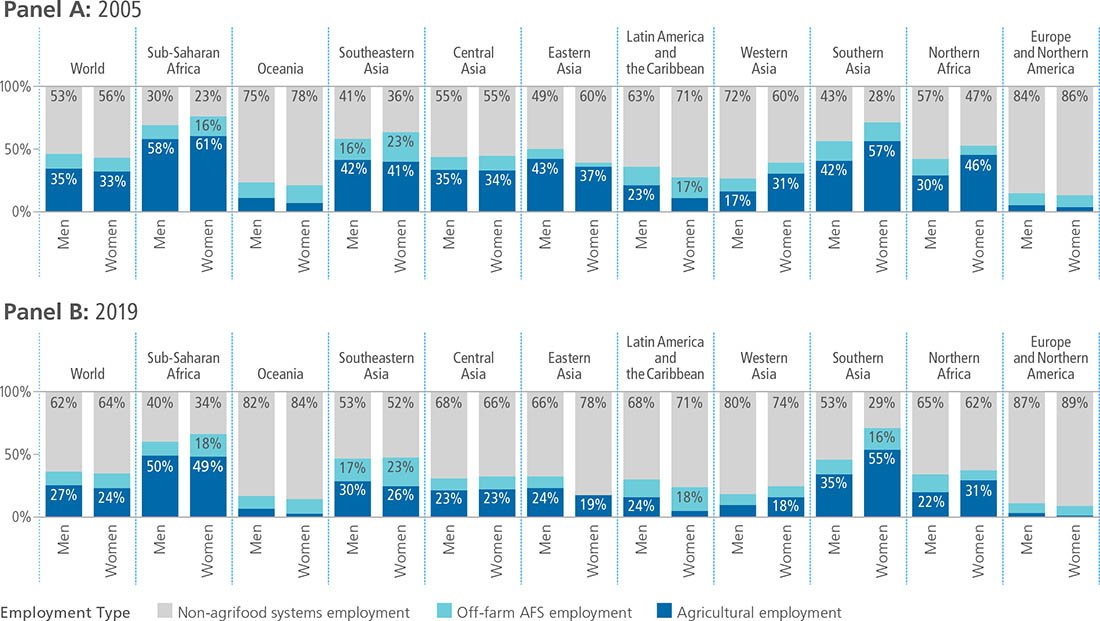
NOTE: The regional and global statistics are weighted by the population of each country.
SOURCE: Costa, V., Piedrahita, N., Mane, E., Davis, B., Slavchevska, V. & Gurbuzer, Y. L. (forthcoming). Women’s employment in agrifood systems. Background paper for The status of women in agrifood systems, 2023. Rome, FAO.
Annex 2. Detailed results on the link between women's empowerment and nutrition
Annex Table 2.1 WEAI & children’s dietary and nutrition outcomes
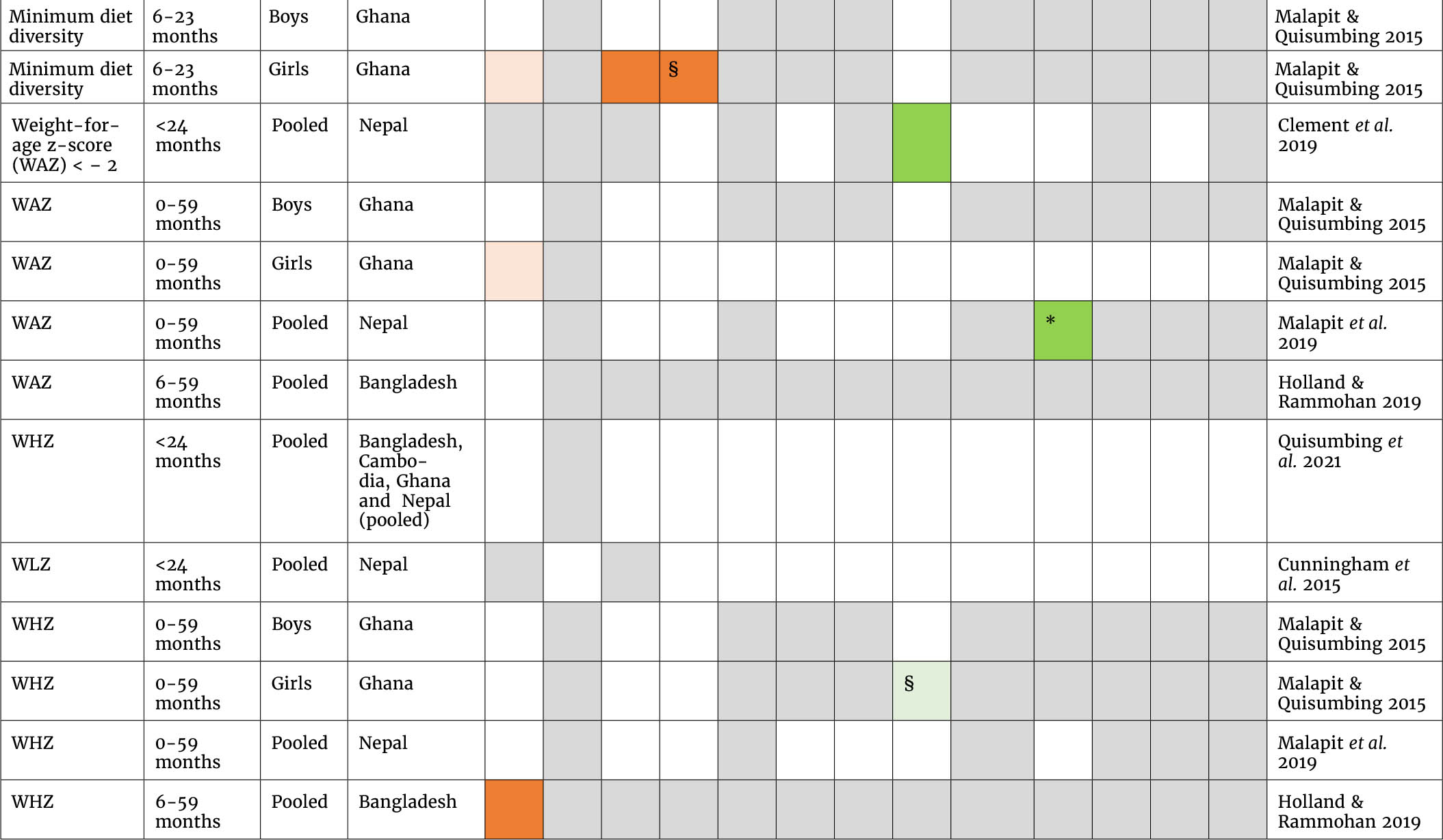
NOTES: § indicates continuous version of indicator; * indicates gap measure. White shading indicates non-significant results; grey shading indicates not measured or included in A-WEAI measure.
DDS – dietary diversity score; HAZ – Height-for-age z-score; WAZ – Weight-for-age z-score; WHZ – Weight-for-height z-score; WLZ – weight for length z-score.

SOURCES:Bonis-Profumo, G., Stacey, N. & Brimblecombe, J. 2021. Measuring women’s empowerment in agriculture, food production, and child and maternal dietary diversity in Timor-Leste.
Food Policy, 102: 102102.
https://doi.org/10.1016/j.foodpol.2021.102102Clement, F., Buisson, M.-C., Leder, S., Balasubramanya, S., Saikia, P., Bastakoti, R., Karki, E. & van Koppen, B. 2019. From women’s empowerment to food security: Revisiting global discourses through a cross-country analysis.
Global Food Security, 23: 160–172.
https://doi.org/10.1016/j.gfs.2019.05.003Cunningham, K., Ploubidis, G.B., Menon, P., Ruel, M., Kadiyala, S., Uauy, R. & Ferguson, E., 2015. Women’s empowerment in agriculture and child nutritional status in rural Nepal.
Public Health Nutrition, 18(17), pp.3134-3145.
https://doi.rg/10.1017/S1368980015000683Holland, C. & Rammohan, A. 2019. Rural women’s empowerment and children’s food and nutrition security in Bangladesh.
World Development, 124: 104648.
https://doi.org/10.1016/j.worlddev.2019.104648Malapit, H. & Quisumbing, A. 2015. What dimensions of women’s empowerment in agriculture matter for nutrition in Ghana?
Food Policy, 52: 54–63.
https://doi.org/10.1016/j.foodpol.2015.02.003Malapit, H., Sraboni, E., Quisumbing, A.R. & Ahmed, A.U. 2019. Intrahousehold empowerment gaps in agriculture and children’s well-being in Bangladesh.
Development Policy Review, 37(2), pp.176-203.
https://doi.org/10.1111/dpr.12374Quisumbing, A., Sproule, K., Martinez, E.M. & Malapit, H. 2021. Do tradeoffs among dimensions of women’s empowerment and nutrition outcomes exist? Evidence from six countries in Africa and Asia.
Food Policy, 100: 102001.
https://doi.org/10.1016/j.foodpol.2020.102001Sraboni, E. & Quisumbing, A. 2018. Women’s empowerment in agriculture and dietary quality across the life course: Evidence from Bangladesh.
Food Policy, 81: 21–36.
https://doi.org/10.1016/j.foodpol.2018.09.001Zereyesus, Y.A., Amanor-Boadu, V., Ross, K.L. and Shanoyan, A. 2017. Does women’s empowerment in agriculture matter for children’s health status? Insights from Northern Ghana.
Social Indicators Research, 132, pp.1265-1280.
https://doi.org/10.1007/s11205-016-1328-z Annex Table 2.2 WEAI & Women’s dietary and nutrition outcomes
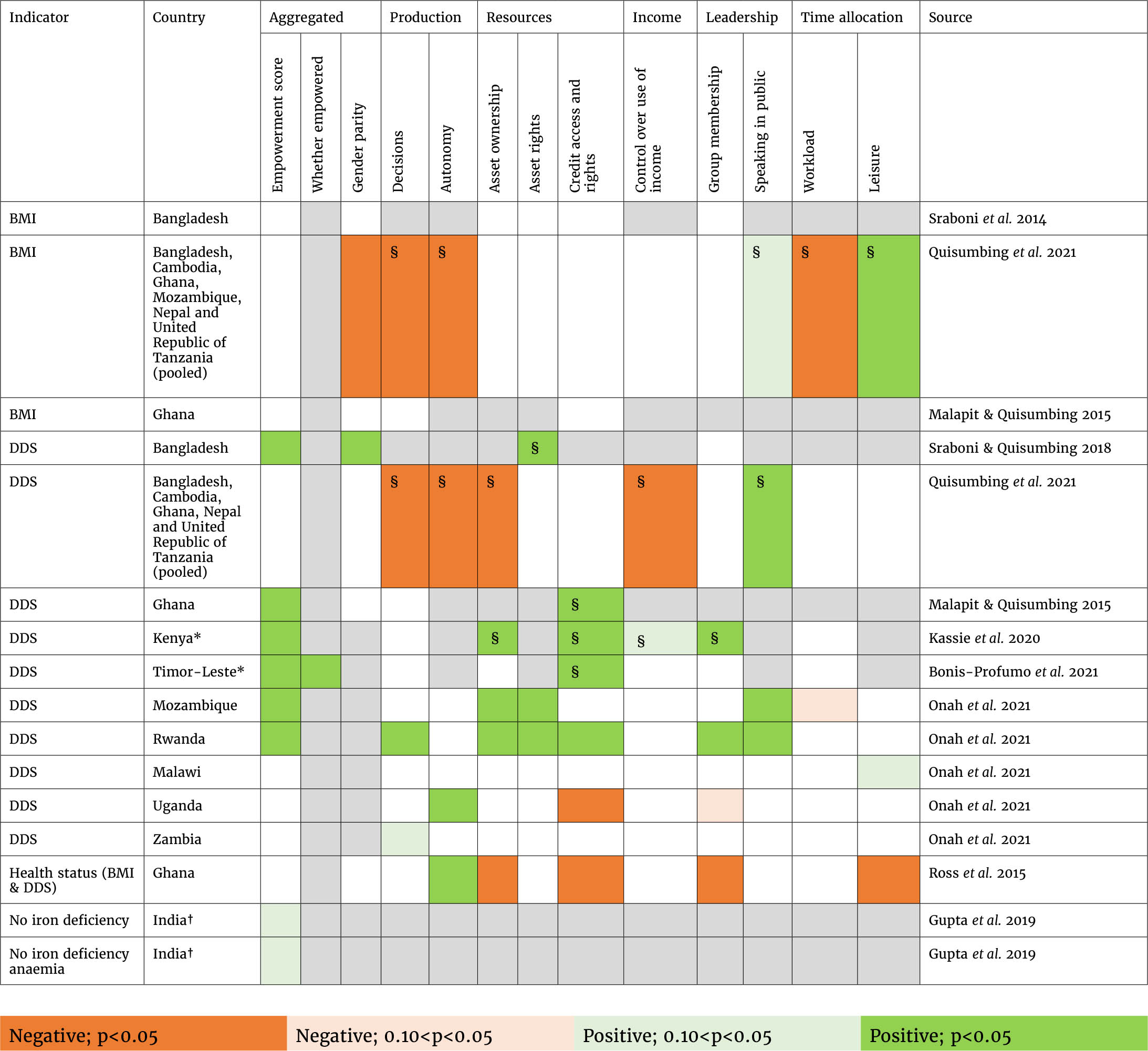
NOTES: § indicates continuous version of indicator; † indicates modified-WEAI version. White shading indicates non-significant results; grey shading indicates not measured or included in A-WEAI measure.
BMI – body mass index; DDS – dietary diversity score.
SOURCES:Bonis-Profumo, G., Stacey, N. & Brimblecombe, J. 2021. Measuring women’s empowerment in agriculture, food production, and child and maternal dietary diversity in Timor-Leste.
Food Policy, 102: 102102.
https://doi.org/10.1016/j.foodpol.2021.102102Gupta, S., Vemireddy, V., Singh, D. & Pingali, P. 2019. Adapting the Women’s Empowerment in Agriculture Index to specific country context: Insights and critiques from fieldwork in India.
Global Food Security, 23, pp.245-255.
https://doi.org/10.1016/j.gfs.2019.09.002Kassie, M., Fisher, M., Muricho, G. & Diiro, G. 2020. Women’s empowerment boosts the gains in dietary diversity from agricultural technology adoption in rural Kenya.
Food Policy, 95: 101957.
https://doi.org/10.1016/j.foodpol.2020.101957Malapit, H. & Quisumbing, A. 2015. What dimensions of women’s empowerment in agriculture matter for nutrition in Ghana?
Food Policy, 52: 54–63.
https://doi.org/10.1016/j.foodpol.2015.02.003Onah, M.N., Horton, S. & Hoddinott, J. 2021. What empowerment indicators are important for food consumption for women? Evidence from 5 sub-Sahara African countries.
PLOS ONE, 16(4): e0250014.
https://doi.org/10.1371/journal.pone.0250014Quisumbing, A., Sproule, K., Martinez, E.M. & Malapit, H. 2021. Do tradeoffs among dimensions of women’s empowerment and nutrition outcomes exist? Evidence from six countries in Africa and Asia.
Food Policy, 100: 102001.
https://doi.org/10.1016/j.foodpol.2020.102001Ross, K.L., Zereyesus, Y., Shanoyan, A. & Amanor-Boadu, V. 2015. The health effects of women empowerment: recent evidence from Northern Ghana.
International Food and Agribusiness Management Review, 18(1030-2016-83056), pp.127-143.
http://dx.doi.org/10.22004/ag.econ.197777Sraboni, E. & Quisumbing, A. 2018. Women’s empowerment in agriculture and dietary quality across the life course: Evidence from Bangladesh.
Food Policy, 81: 21–36.
https://doi.org/10.1016/j.foodpol.2018.09.001Sraboni, E., Malapit, H., Quisumbing, A. & Ahmed, A.U. 2014. Women’s empowerment in agriculture: What role for food security in Bangladesh?
World Development, 61: 11–52.
https://doi.org/10.1016/j.worlddev.2014.03.025 Annex Table 2.3 WEAI & men’s dietary and nutrition outcomes
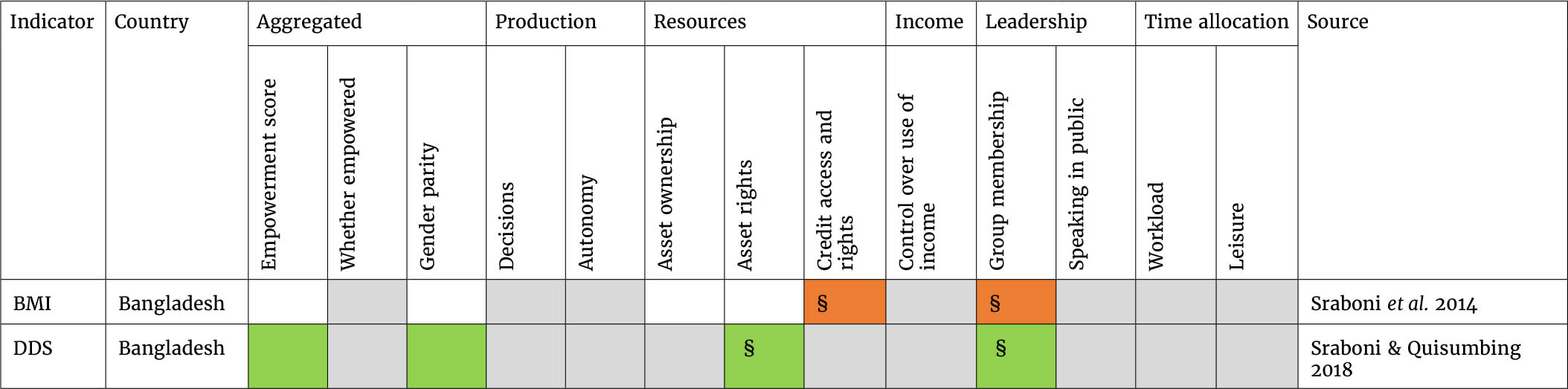
NOTES: § indicates continuous version of indicator. White shading indicates non-significant results; grey shading indicates not measured or included in A-WEAI measure.
BMI – body mass index; DDS – dietary diversity score.
SOURCES:Sraboni, E. & Quisumbing, A. 2018. Women’s empowerment in agriculture and dietary quality across the life course: Evidence from Bangladesh.
Food Policy, 81: 21–36.
https://doi.org/10.1016/j.foodpol.2018.09.001Sraboni, E., Malapit, H., Quisumbing, A.
& Ahmed, A.U. 2014. Women’s empowerment in agriculture: What role for food security in Bangladesh?
World Development, 61: 11–52.
https://doi.org/10.1016/j.worlddev.2014.03.025 Annex Table 2.4 WEAI & household dietary and nutrition outcomes
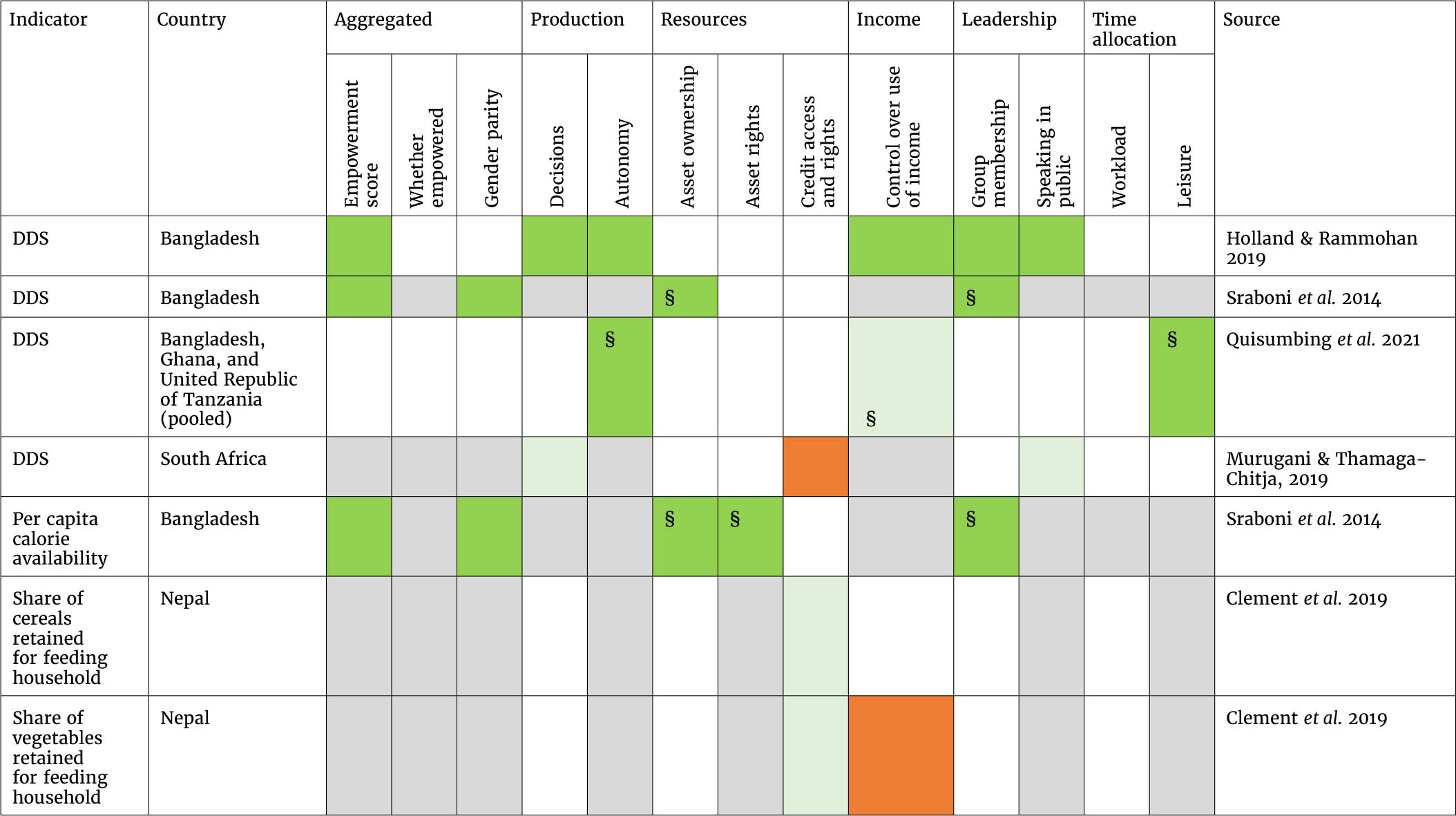
NOTES: § indicates continuous version of indicator. White shading indicates non-significant results; grey shading indicates not measured or included in A-WEAI measure.
DDS – dietary diversity score.
SOURCES:Clement, F., Buisson, M.-C., Leder, S., Balasubramanya, S., Saikia, P., Bastakoti, R., Karki, E. & van Koppen, B. 2019. From women’s empowerment to food security: Revisiting global discourses through a cross-country analysis.
Global Food Security, 23: 160–172.
https://doi.org/10.1016/j.gfs.2019.05.0036 1Holland, C. & Rammohan, A. 2019. Rural women’s empowerment and children’s food and nutrition security in Bangladesh.
World Development, 124: 104648.
https://doi.org/10.1016/j.worlddev.2019.104648Murugani, V.G. & Thamaga-Chitja, J.M. 2019. How does women’s empowerment in agriculture affect household food security and dietary diversity? The case of rural irrigation schemes in Limpopo Province, South Africa.
Agrekon, 58(3): 308–323.
https://doi.org/10.1080/03031853.2019.1610976Quisumbing, A., Sproule, K., Martinez, E.M. & Malapit, H. 2021. Do tradeoffs among dimensions of women’s empowerment and nutrition outcomes exist? Evidence from six countries in Africa and Asia.
Food Policy, 100: 102001.
https://doi.org/10.1016/j.foodpol.2020.102001Sraboni, E., Malapit, H., Quisumbing, A. & Ahmed, A.U. 2014. Women’s empowerment in agriculture: What role for food security in Bangladesh?
World Development, 61: 11–52.
https://doi.org/10.1016/j.worlddev.2014.03.025 Annex 3. Methodology for estimating the benefits of closing the gender gaps in farm productivity and wages
This annex describes the methodology and the data sources used to estimate the potential impact of closing the gender gaps in farm productivity and wages in agrifood systems on gross domestic product (GDP) and food insecurity. The approach uses the estimations presented in Chapter 2 on the gender gaps in farm productivity and wage gaps in agriculture to measure the income gains from reducing those gaps and then simulates the potential impact on food insecurity using income elasticities derived from the food insecurity Tobit model described in Box 1.1 in Chapter 1.
The equations, assumptions and data sources presented below provide a conservative measure of the impacts because we do not assess the multiplier and spillover effects of reducing the gender income gaps on the whole economy. The estimations are conducted separately for countries aggregated by income groups. We show that closing the gaps in farm productivity and wages in agrifood systems would increase global GDP by at least 1 percent (or nearly USD 1 trillion). This would reduce global food insecurity by at least 2 percentage points, reducing the number of food-insecure people by 45 million.
Farm productivity gap model
GDP, following a simplified value-added approach, is defined as the sum of the value added of the agricultural sector,YA, and non-agricultural sectors,YN:

Using this simple accounting definition, one can show that, as a result of an expansion of agricultural value added, GDP would grow according to the expression:
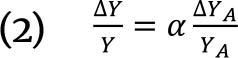
where the share
defines the portion of national GDP that is due to agricultural production.
On the other hand, agricultural value added can be decomposed as the product of average agricultural yields per land area (YA/A) and the total area devoted to agricultural production, A:
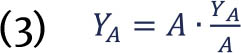
This total agricultural output can be separated into the output produced in male farms
and the output produced in female farms
:

Using this accounting definition, total agricultural production can be decomposed using yields, according to the expression:

where the yields
are different for male (M) and female farms (F). Given that area under female farms and area under male farms must equal the total area,
, total agricultural production can be decomposed, using differentiated yields by gender as:

where β is the share of the total agricultural land area held by female farms,
. Using expression (5), the impact on agricultural output of increasing yields in female farms is:
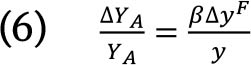
For simulation purposes we assume that the gender yield gap is closed and that female farm yields grow according to the expression:

Using expressions (2), (6) and (7), we can express the impact on national GDP of closing the gender yield gap as:
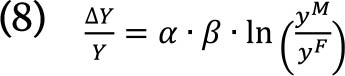
The overall ratio of farmland managed by female farmers to male farmers, β, is obtained by multiplying the ratio of female- to male-managed agricultural holdings
, as reported by the agricultural censuses, with the ratio of the average farm size of female-managed farms to male-managed farms,
, estimated using household surveys and approximately equal to 0.69.
Annex Table 3.1 The impact of closing the farm productivity gender gap on gross domestic product and food insecurity
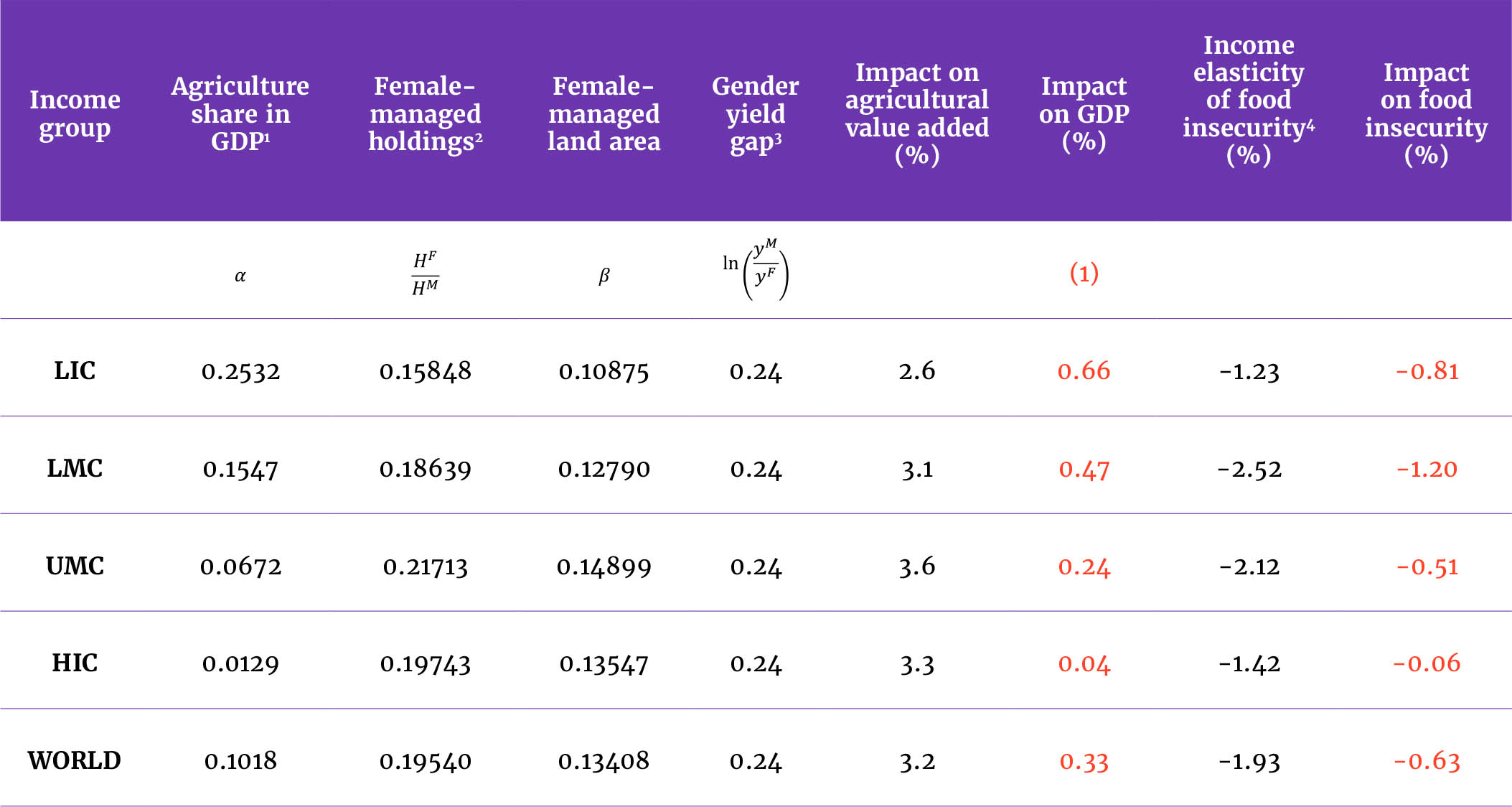
NOTES: LIC – low-income countries; LMC – lower-middle-income countries; UMC – upper-middle-income countries; HIC – high-income countries
Wage gap model
GDP, following the income approach, can also be understood as national income distributed to workers (wL), returns to capital owners (rK) and taxes (T):

If only wages expand in the economy, then GDP would grow according to:
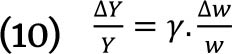
where γ is the share of labour income in national GDP,
.
The total labour bill can be decomposed into income earned by males (M) and that earned by females (F):

Using expressions (11) and (10), we can express the impact of raising female wages on national GDP as:
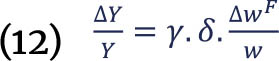
where δ is the share of female employment in total national employment,
. Hence, the effect of eliminating the gender wage gap on national GDP would be obtained using expression (10) and the expected percentage wage gain:
.
If we focus on raising female wages in agrifood systems (AFS) only, equation (12) becomes:

Given the data sources at hand, we have information on the share of female employment in agrifood systems, δAFS from Chapter 2, while in the absence of a precise measure of gender wage gaps in agrifood systems
, this gap is assumed to be equivalent to the wage gaps found in the agricultural sector. The contribution of the AFS wage bill to national GDP,
, is a bit more difficult to obtain with the available data. Hence, we approximate it with the following formula:

which implicitly assumes that AFS wages are the same as the wages in other sectors. Thus, the final equation to estimate the impact of closing the gender wage gaps in agrifood systems on GDP becomes:

Annex Table 3.2 The impact of closing the gender gap in agrifood systems on gross domestic product and food insecurity
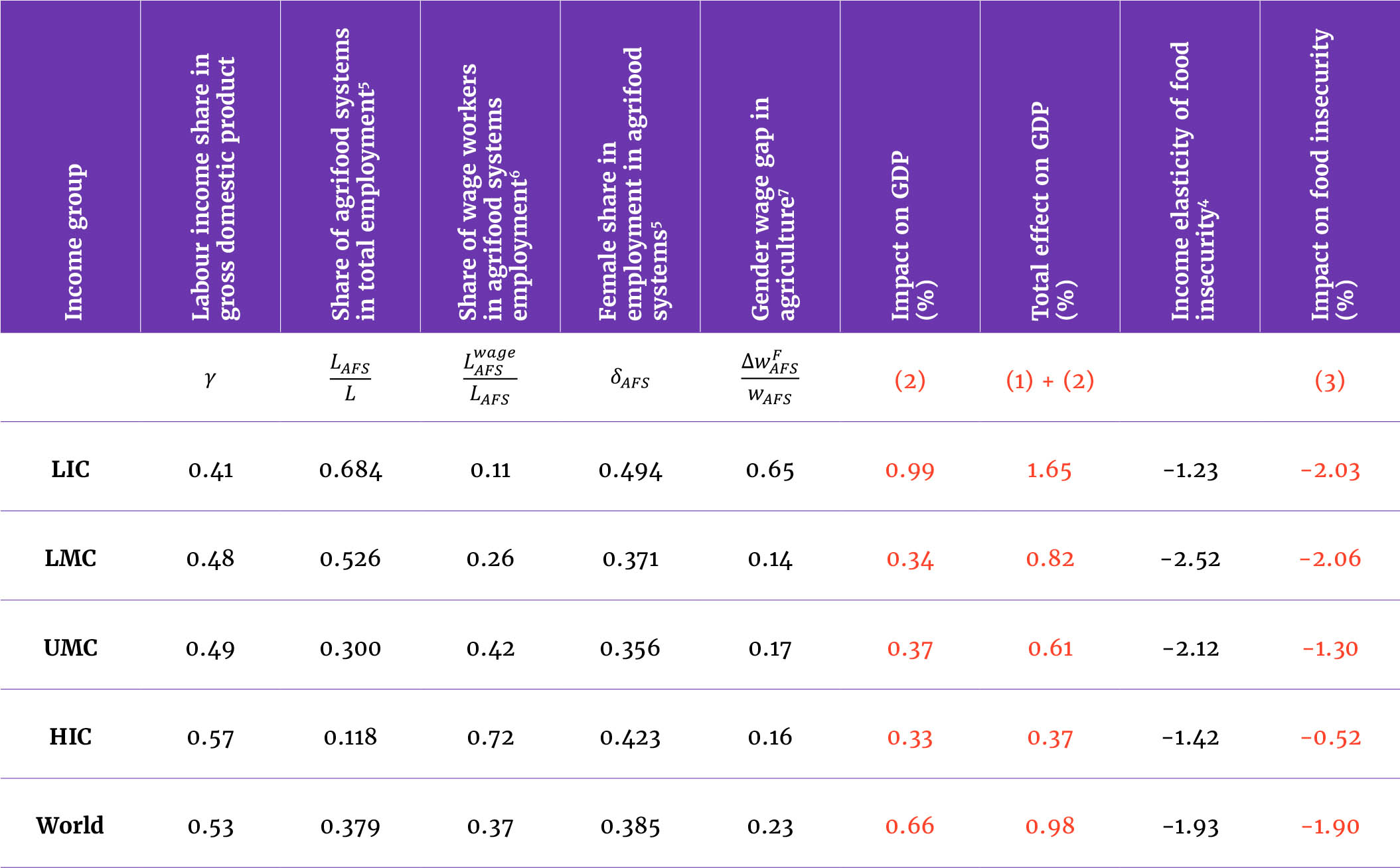
NOTES: LIC – low-income countries; LMC – lower-middle-income countries; UMC – upper-middle-income countries; HIC – high-income countries
2. Share of holdings operated by civil persons, female. Source:
FAO. 2023. Structural data from agricultural censuses. In:
Food and Agriculture Organization of the United Nations, FAOSTAT. Rome. Cited 22 March 2023.
https://www.fao.org/faostat/en/#data/WCAD3. Anríquez, G., Quiñonez, F. & Foster, W. (forthcoming). Levelling the farm fields, A cross-country study of the determinants of gender-based yield gaps. Background paper for The status of women in agrifood systems, 2023. Rome, FAO. This study measures farm productivity gap drawing on national survey data from 11 countries in Africa, Latin America and the Caribbean and Asia: Bangladesh Household Income and Expenditure Survey 2010; Ethiopian Socioeconomic Survey 2018/19; Ghana Living Standards Survey 2012/13; Guatemala Encuesta Nacional de Condiciones de Vida 2014; Cambodia Socio Economic Survey 2009; Malawi Integrated Household Survey 2017; Nicaragua Encuesta de Medición de Nivel de Vida 2014; Pakistan Social And Living Standards, Measurement Survey 2013/14; Peru Encuesta Nacional de Hogares 2019; Uganda National Panel Survey 2016; Vietnam Household Living Standard Survey 2010.
4. The income elasticities of food insecurity are estimated using the Tobit food insecurity model described in Mane, E., Macchioni, G.A., Cafiero, C. & Viviani, S. (forthcoming). Why are women more food insecure than men? Exploring socioeconomic drivers and the role of COVID-19 in widening the global gender gap. Background paper for The status of women in agrifood systems, 2023. rome, FAO. The model uses food insecurity estimates, income and other individual-level variables to estimate income elasticities for 121 countries during the period 2014-2021. Then, simple country averages are implemented to calculate income elasticities for each income group.
5. Own computations using 2019 data of the dataset constructed by
Davis, B., Mane, E., Gurbuzer, L.Y., Caivano, G., Piedrahita, N., Schneider, K., Azhar, N. et al. 2023.
Estimating global and country-level employment in agrifood systems. FAO Statistics Working Paper Series, No. 23-34. Rome, FAO.
https://doi.org/10.4060/cc4337en6. The share was computed based on data from ILO Harmonized Microdata (
https://ilostat.ilo.org/). These were used to compute the share of wage workers in agrifood systems in each country (number of wage workers in agrifood systems/number of total persons working in agrifood systems). Data were available for 101 countries.
7. The wage gap estimate was obtained from Benali, M., Slavchevska, V., Davis, B., Piedrahita, N., Sitko, N., Nico, G. & Azzarri, C. (forthcoming). Gender pay gaps among agriculture and non-agriculture wage workers: a cross-country examination. Background paper for The status of women in agrifood systems, 2023. Rome, FAO.
Annex 4. Methodology for estimating the benefits of reaching small-scale producers with development interventions that focus on womens's empowerment
The International Fund for Agricultural Development (IFAD) measures the impacts of the interventions it finances by systematically conducting impact assessments on a sample of projects selected from those closing during each replenishment cycle. Between 2019 and 2021, IFAD conducted such impact assessments on 24 out of 96 projects that closed during that period, which corresponds to the eleventh Replenishment of IFAD’s Resources.1 The sample covers USD 3.1 billion in IFAD investments and represents an overall investment of USD 7.1 billion (including cofinancing).2
The methodology used consists of ex post quasi-experimental impact assessments and allows the estimation of the impacts achieved by projects on key indicators of interest.
Meta-analysis is then conducted on the estimated impacts of the 24 projects to estimate mean effect sizes.3 Once it is established that the sample of projects is not statistically significantly different from the universe of projects that closed during the same period, the mean effect sizes represent attributable impacts of interventions. They can be interpreted as percentage changes in the indicators estimated for the beneficiaries (or treatment group) over the comparison group. Once the mean effect sizes are estimated, a projection exercise is implemented to compute aggregate impacts of the entire portfolio of projects that have closed in the reference period.
Following IFAD’s approach, we conduct an exercise to estimate results obtainable in the case in which all projects had a women’s empowerment focus. To this purpose we identify treatment and comparison groups by distinguishing two types of projects in our sample: projects that improve women’s decision-making power over the use of income and/or resources versus projects that do not contribute to such improvement.4 Once this classification is done, we replicate the meta-analysis and projection, which, as reported in IFAD (2022),2 is based on one important assumption related to the distribution of impacts. It is assumed that the estimated impacts are normally distributed across the entire population of beneficiaries and have the same means and standard deviations as the estimated impacts (i.e. mean effect sizes). As a next step we calculate the total number of beneficiaries who have achieved results above the following targets:
- At least 10 percent increase in income;
- At least 20 percent increase in production;
- At least 20 percent improved market access;
- At least 20 percent improved resilience;
- At least 10 percent more food secure;
- At least 10 percent with improved nutrition (diversified diet).
We: 1) randomly draw a normal distribution of impacts (with an associated mean and standard deviation as empirically estimated from the meta-analysis) for 112 million people, which corresponds to the total number of beneficiaries reached by the 96 IFAD-funded projects; and 2) count the number of people that have experienced an increase that exceeds the thresholds for key outcome indicators. The results are summarized in Annex Table 4.1.
Annex Table 4.1 Projection results for projects with a women’s empowerment focus versus standard projects with no women’s empowerment focus.
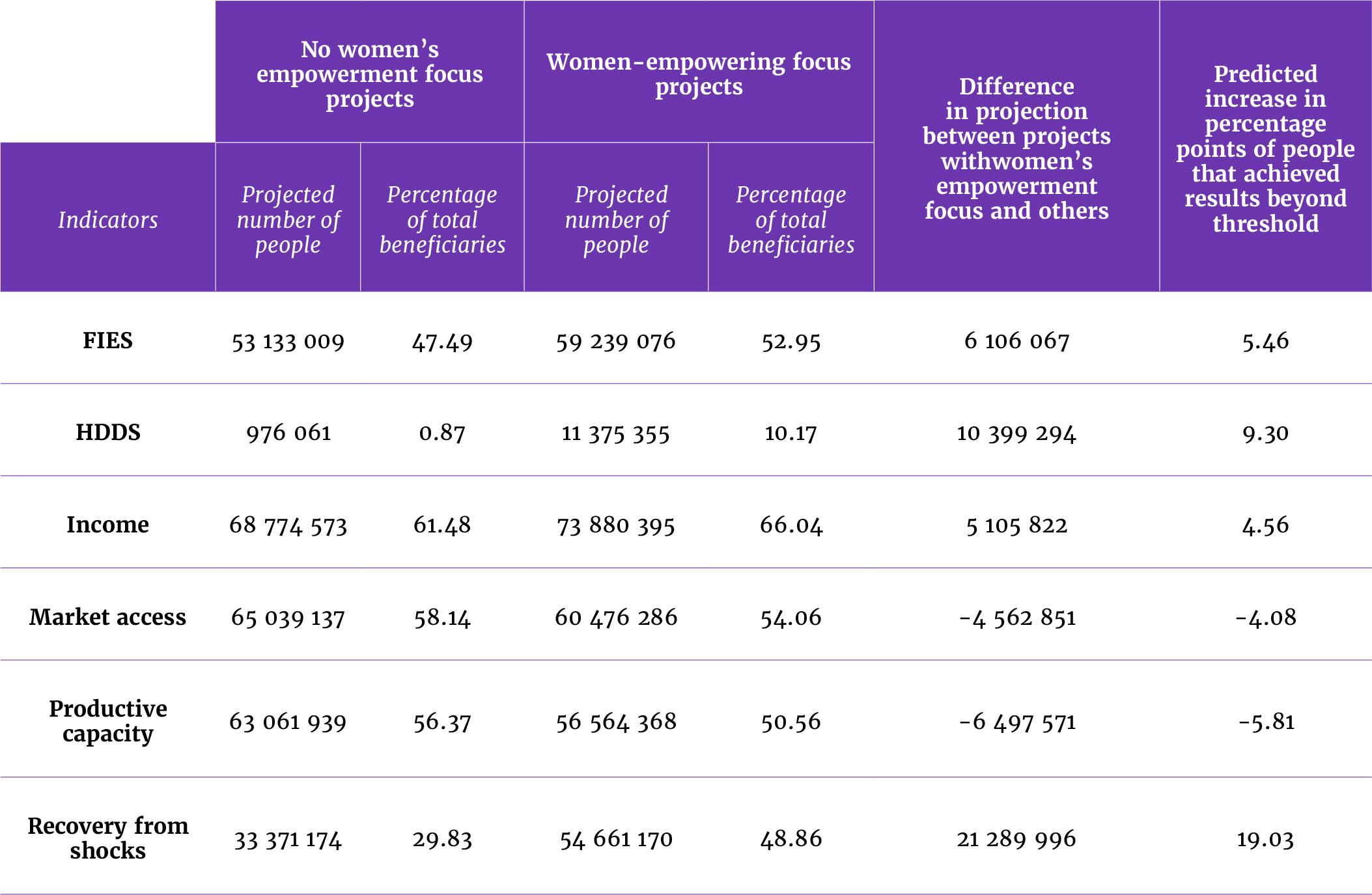
NOTES: FIES – Food Insecurity Experience Scale; HDDS – Household Dietary Diversity Score. The numbers in
Annex Table 4.1 refer to the number of people that achieved improvement in indicators beyond the thresholds listed above.
These results suggest that with respect to income, food insecurity measured by FIES, household dietary diversity measured by HDDS and recovery from shocks, every person – men and women – benefits in a significant fashion from projects where women enjoy greater decision-making power. On the other hand, and in line with other findings, agricultural productivity and access to markets is lower for people in the group of non-women’s empowering projects. This is a reasonable result given that most of the successful gains resulted from agribusiness opportunities, fishery and/or livestock and dairy projects rather than from primary agricultural production.
These findings also suggest that interventions that are comprehensive and multifocused on improving households’ benefits while placing emphasis on the role played by women are more successful in many domains, although there is still scope for improvements. For example, productivity and access to market require more focused investments towards production-oriented goals.
Donors already investing in agriculture and rural development projects can thus enhance people’s livelihoods by focusing on approaches that improve women’s well-being by enhancing their empowerment while simultaneously increasing incomes, dietary diversity, food security and resilience for households.